Hiding the Magic
The hidden cost of ChatGPT, an Information Design Journal, and the MusicCaps dataset
Hi,
In preparation for this newsletter, I broke a small personal rule: I logged back into Twitter. Historically, Twitter was my main information feed for finding interesting links for Data Curious. After the Musk takeover I reached a breaking point and jumped ship to Mastodon (vis.social server). I’m grateful that a small but not insignificant number of people I admire from my Twitter-sphere also did the same.
That being said, Mastodon hasn’t quiet rivaled the level of discovery achieved on Twitter. On the bright side, it’s not (as of now) a soul-sucking portal designed to encourage outrage and dunking on people. So pros and cons.
But every once in a while (like yesterday), I’ll pop my head back in to see if there’s anything in the data + tech world that might be inspiring. I’m not sure if I regret it yet or not. One thing that really stood out as different: the AI hype is everywhere.
I don’t typically write about AI. It’s not my field of interest or expertise. And I’m not going to offer much editorial on it in this letter (only a bit). But I will say that I recently finished reading Atlas of AI by Kate Crawford, which helped me understand the wider scope of where we are now and how we got here. Highly recommend it for a critical reading of machine learning technologies (i.e. AI, minus the marketing hype).
That being said, I will be featuring some AI related content here (but not at all for promotional purposes…quite the opposite in fact).
If you have any other recommendations for critical analysis of AI, let me know in the comments below or by replying to this email.
Read
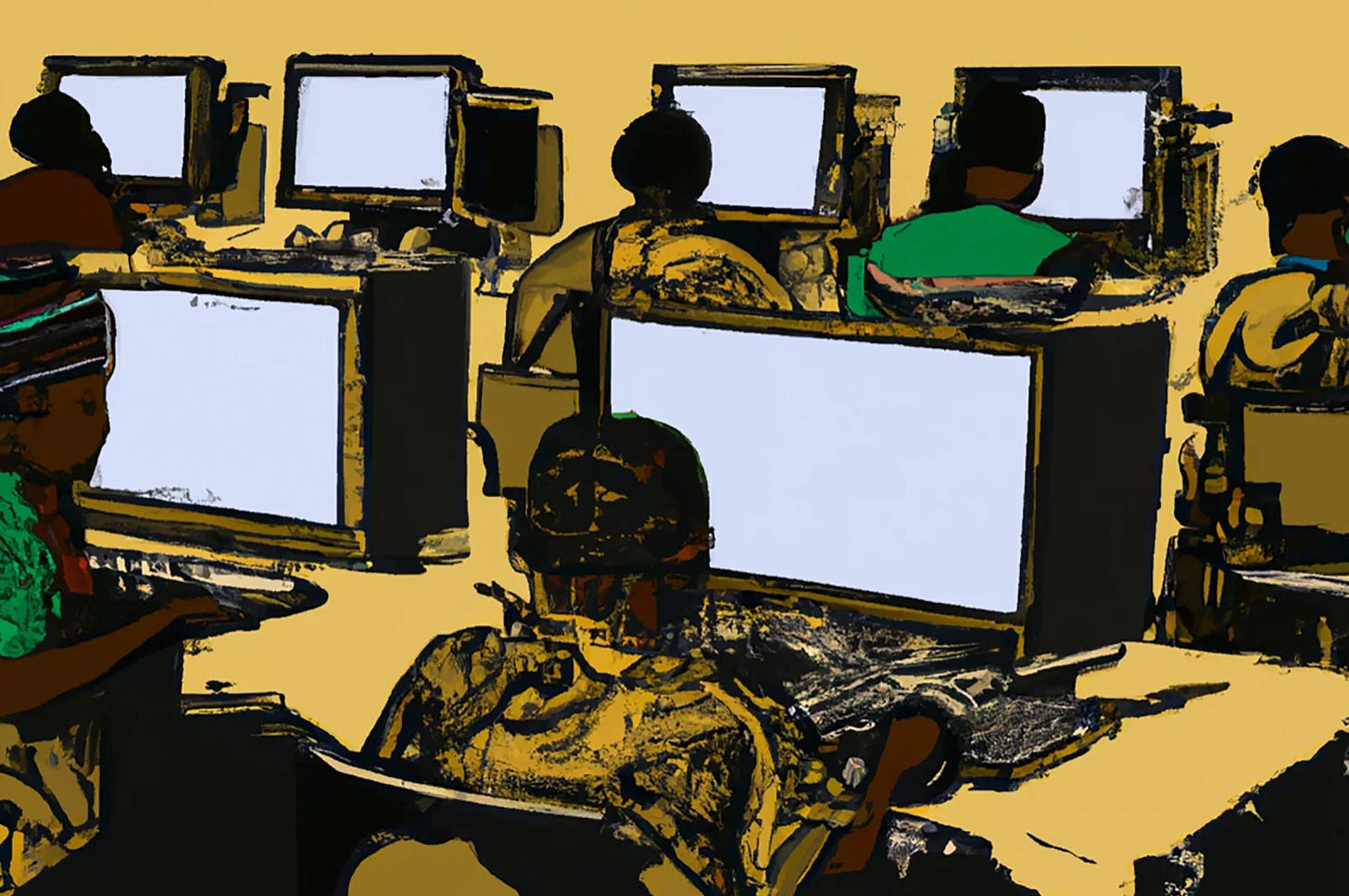
OpenAI Used Kenyan Workers on Less Than $2 Per Hour to Make ChatGPT Less Toxic
Ok, I know I said that I wouldn’t write much about AI…but this story came across my feed last week and the timing felt eerily prescient. I had just been reading in Atlas of AI how fundamentally, AI technology is one of extraction. Extracting data in the form of scraping huge amounts of information from the web. Extracting valuable minerals from the Earth to fuel the huge amount of computing power needed to run the models. And extracting human labor (as demonstrated in this investigative TIME piece) by outsourcing content moderation and data labeling at the lowest possible costs.
Users of ChatGPT see exactly what OpenAI want them to see: the instant magic of a machine answering your questions reasonably. But as the piece shows, much of the work is not magic: it’s human exploitation.
Explore (but mostly read)
Information Design Journal (Issue 1, Volume 27) is now Open Access
I was unfamiliar with this publication until last week, when I saw someone announce that the first issue of this year was just made Open Access (all articles available online). In my experience, this is rare for academic style journals. From a quick skim, I’m eager to have a closer look, especially with titles such as “A dynamic topography for visualizing time and space in fictional literary texts”.

Data


MusicLM: Generating Music From Text (Dataset)
Admittedly, I was impressed by MusicLM when I first heard it. MusicLM is the newest text-to-music generational model on the block from Google Research. But even more interesting to me was (as mentioned by Tero in the tweet above) the fact that the authors open sourced the training dataset: over 5 thousand music examples with text labels attached. Could be some interesting source material to experiment with later, for both machine learning and visualization purposes. The MusicCaps dataset is on Kaggle here and the research paper as a PDF is here.